Research
Published 11 October 2022Ada Lovelace day profiles 2022
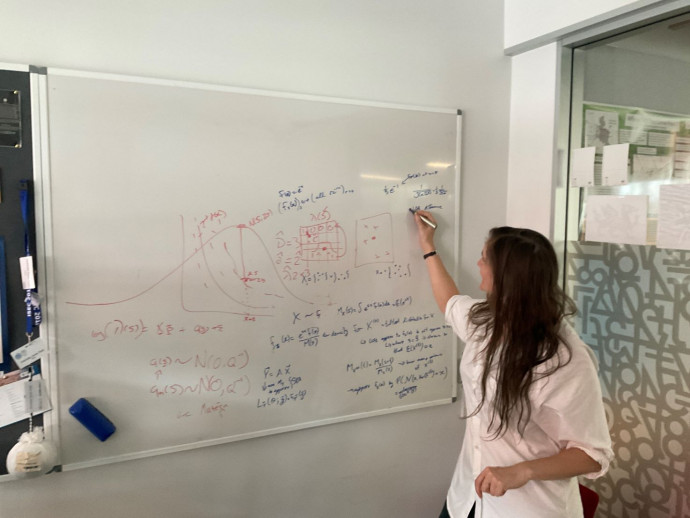
In honour of Ada Lovelace Day 2022 (Rātū 11 Whiringa-a-Nuku Tuesday 11 October), we are featuring the work of three recent Marsden Fund wāhine researchers who are following in Ada’s mathematical footsteps. Ka rawe!
Dr Jodie Hunter
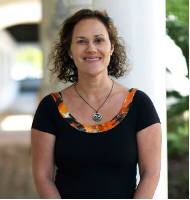
Dr Jodie Hunter (supplied)
Dr Jodie Hunter is PI on Marsden Fund Fast-Start MAU1910 “Exploring of Pāsifika funds of knowledge in mathematics.”
Associate Professor Jodie Hunter is affiliated with the Institute of Education at Te Kunenga Ki Pūrehuroa Massey University, Albany. In 2019, Associate Professor Hunter received Fast-Start funding to explore culturally embedded ways of knowing and successful mathematical experiences of Pāsifika learners outside of school, in their everyday settings in the home and community.
Equity in schooling requires educators to develop understandings of the identities of diverse learners and their ‘funds of knowledge.’ Aotearoa has one the largest groups of Pāsifika people in the Western world. Our Pāsifika communities are woven from many threads of diverse ethnicities, nationalities, languages, and cultures. However, this cultural knowledge is currently excluded from the classroom. Associate Professor Hunter is working with Pāsifika students aged from 7–15 and their families to document their school experiences with mathematics, aiming to raise awareness of the strengths of Pāsifika learners and address the current equity issues in education.
Dr Charlotte Jones-Todd
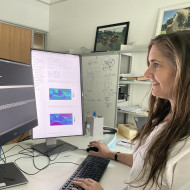
Dr Charlotte Jones-Todd
Dr Charlotte Jones-Todd is the PI on Marsden Fund Fast-Start UOA2121 “Rejuvenating the role of random fields in modelling spatiotemporal point patterns: A new era of point process models.”
Dr Charlotte Jones-Todd is a Lecturer in Statistics at Waipapa Taumata Rau University of Auckland. In 2021, Dr Jones-Todd was awarded a Marsden Fund Fast-Start to better understand how events cluster in time and space. This work will help explain the underlying mechanisms behind real-world phenomena such as tweets going viral, neighbourhood crime waves, and earthquake aftershocks.
The complex mechanism behind any real-world chain of events is not easily measured or perceived. In this research, Dr Jones-Todd will develop new statistical frameworks for modelling events. The models created will incorporate self-exciting behaviours, which are when the presence of one event makes another event more likely. The combination of both event interrelationships and self-exciting behaviour will make the models practical and adaptable. The mathematical frameworks created over the course of this project could see use in a range of different fields from ecology to epidemiology and everywhere in between.
Professor Bing Xue
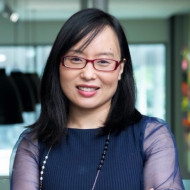
Professor Bing Xue (supplied)
Professor Bing Xue is PI on Marsden Fund Standard VUW1914 “Evolutionary Automated Design of Deep Convolutional Neural Networks for Image Classification.”
Professor Bing Xue, of the School of Engineering and Computer Science at Te Herenga Waka Victoria University of Wellington received a Marsden Fund Standard grant in 2019 to use deep learning algorithms to address current challenges in identifying patterns in images for many real-world applications.
Deep machine learning techniques, particularly deep convolutional neural networks (DCNNs), are very successful in image classification. However, almost all state-of-the-art DCNNs are manually designed, requiring the ongoing support of rich experience and expensive expertise in DCNNs applied to a specific problem. These DCNNS are too complex to interpret, limiting their practical utility. Automated design of optimal DCNNs would address these issues, but this is very challenging because optimal architecture is highly complex and problem dependent. Professor Xue’s project is developing an evolutionary computation-based approach to automated design of DCNN architectures. These new models will improve the classification accuracy, speed, simplicity, and interpretability of the learned DCNNs. These novel DCNNs will have numerous applications in security, self-driving vehicles, medicine and beyond.